B - Trees ( Balanced Search Trees )
A B-tree is a tree data structure that keeps data sorted and allows searches, insertions, and deletions in logarithmic amortized time. Unlike self-balancing binary search trees, it is optimized for systems that read and write large blocks of data. It is most commonly used in database and file systems.
Properties
- All data is stored in leaves, in a sorted order.
- The root is a leaf or has between 2 and M children
- Each interior node is at least half full -- has between éM/2ù and M children
- All leaves are at the same depth
- All leaves are at least half full -- store between éL/2ù and L data items
Height of a B tree
Basic operations on B-Trees
Searching a B-tree
Searching a B-tree is much like searching a binary search tree, except that instead of making a binary, or "two-way," branching decision at each node, we make a multiway branching decision according to the number of the node's children. More precisely, at each internal node x, we make an (n[x] + 1)-way branching decision.
B-TREE-SEARCH(x, k)1 i = 1
2 while i ≤ n[x] and k ≥ keyi[x]
3 do i = i + 1
4 if i ≤ n[x] and k = keyi[x]
5 then return (x, i)
6 if leaf [x]
7 then return NIL
8 else DISK-READ(ci[x])
9 return B-TREE-SEARCH(ci[x], k)
B-Tree createB-TREE-CREATE(T)1 x = ALLOCATE-NODE()
2 leaf[x] = TRUE
3 n[x] = 0
4 DISK-WRITE(x)
5 root[T] = x
B-TREE-CREATE requires O(1) disk operations and O(1) CPU time.
Splitting a node with t = 4. Node y is split into two nodes, y and z, and the median key S of y is moved up into y's parent.
B-Tree Insert
Insertion:
At the leaf node level, insertions are made. The following algorithm must be followed to place an item into B Tree.
- Navigate the B Tree until you find the suitable leaf node to insert the node.
- If there are fewer than m-1 keys in the leaf node, insert the element in ascending order.
- Else, it should proceed to the next step if the leaf node contains m-1 keys.
- In the rising sequence of elements, add the new element.
- In the middle, divide the node into two nodes.
- The median element should be pushed up to its parent node.
- If the parent node has the same m-1 number of keys as the child node, split it.
Figure: Splitting the root with t = 4. Root node r is split in two, and a new root node s is created. The new root contains the median key of r and has the two halves of r as children. The B-tree grows in height by one when the root is split.
B-TREE-INSERT(T,k)1 r = root[T]
2 if n[r] = 2t - 1
3 then s = ALLOCATE-NODE()
4 root[T] = s
5 leaf[s] = FALSE
6 n[s] = 0
7 c1[s] = r
8 B-TREE-SPLIT-CHILD(s,1,r)
9 B-TREE-INSERT-NONFULL(s,k)
10 else B-TREE-INSERT-NONFULL(r,k)
B-TREE-INSERT-NONFULL(x,k)1 i = n[x]
2 if leaf[x]
3 then while i ≥ 1 and k < keyi[x]
4 do keyi+1 [x] = keyi[x]
5 i = i - 1
6 keyi+1[x] = k
7 n[x] = n[x] + 1
8 DISK-WRITE(x)
9 else while i ≥ 1 and k < keyi[x]
10 do i = i - 1
11 i = i + 1
12 DISK-READ(ci[x])
13 if n[ci[x]] = 2t - 1
14 then B-TREE-SPLIT-CHILD(x,i,ci[x])
15 if k > keyi[x]
16 then i = i + 1
17 B-TREE-INSERT-NONFULL(ci[x],k)
Example:
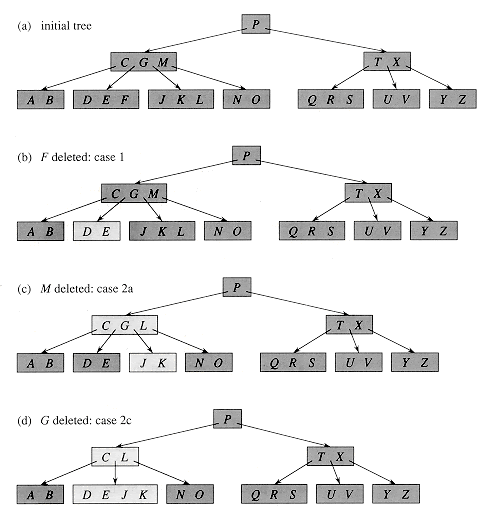
At the leaf nodes, deletion is also conducted. It can be a leaf node or an inside node that must be destroyed. To delete a node from a B tree, use the following algorithm.
- Find the position of the leaf node.
- If the leaf node has more than m/2 keys, delete the required key from the node.
- If the leaf node lacks m/2 keys, fill in the gaps with the eighth or left sibling element.
- If the left sibling has more than m/2 elements, shift the intervening element down to the node where the key is deleted and push the largest element up to its parent.
- If the right sibling has more than m/2 items, shift the intervening element down to the node where the key is deleted and push the smallest element up to the parent.
- Create a new leaf node by merging two leaf nodes and the parent node's intervening element if neither sibling has more than m/2 elements.
- If the parent has less than m/2 nodes, repeat the operation on the parent as well.
If the node to be destroyed is an internal node, its in-order successor or predecessor should be used instead. Because the successor or predecessor will always be on the leaf node, the operation will be similar to deleting the node from the leaf node.
Example:
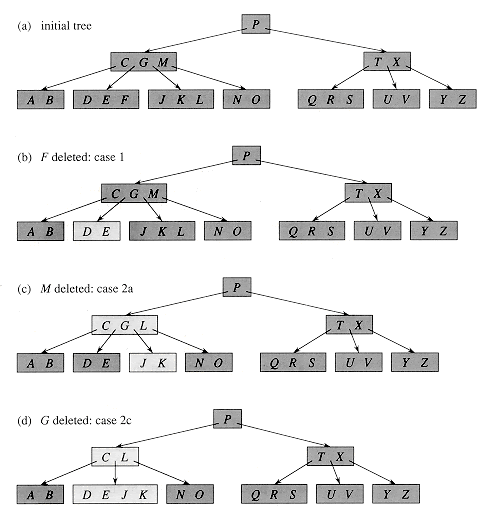
source: staff.ustc.edu.cn
Applications
- Because accessing values held in a vast database saved on a disc is a very time-consuming activity, the B tree is used to index the data and enable fast access to the actual data stored on the discs.
- In the worst situation, searching an unindexed and unsorted database with n key values takes O(n) time. In the worst-case scenario, if we use B Tree to index this database, it will be searched in O(log n) time.
- A B-tree, in particular:
- keeps keys sorted for sequential traversal and uses a hierarchical index to reduce disc reads
- insertions and deletions are sped up by using partially filled blocks.
- a recursive algorithm that keeps the index balanced
Some solved question
Create B-tree of order 5 from the following lists of data items: 20, 30, 35, 85, 10, 55, 60, 25, 5, 65, 70, 75, 15, 40, 50, 80, 45.
0 Comments